


Back to Blog
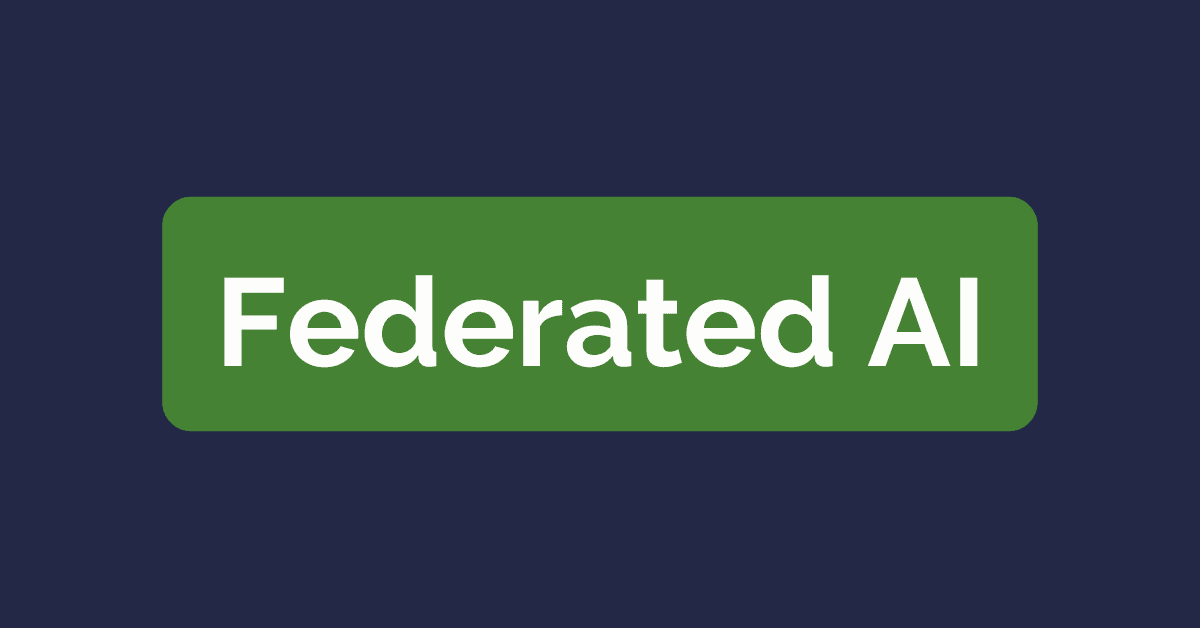
What is Federated AI, and How Is It Shaping the Future of Building Energy Management?
Federated AI is a transformative approach to machine learning that’s gaining traction across industries, especially in fields that require secure, scalable data analysis. Within building energy management, Federated AI is a game changer, allowing systems like Optimise-AI’s Predict and Optimise tools to learn from multiple buildings’ energy data without needing centralised storage. This decentralisation opens new possibilities for energy management, all while preserving data privacy and enhancing adaptability. But what exactly is Federated AI, and why is it so valuable for creating energy-efficient, sustainable buildings?
What Is Federated AI?
Federated AI, also known as Federated Learning (FL), is a machine learning framework where multiple devices or data sources collaborate to train an AI model, all while keeping data localised. Instead of gathering all data on a central server, Federated AI enables individual devices or nodes to learn independently and share only their learned insights (model parameters) with a central model.
For example, imagine multiple buildings, each equipped with an HVAC system, contributing anonymised insights about energy consumption patterns without transferring actual data. Each building "learns" on its own, and only the model updates are shared to improve overall system accuracy. This approach allows learning from diverse data sources while protecting each source’s privacy and confidentiality.
Why Is Federated AI Valuable for Energy Management?
Building energy optimisation often requires vast amounts of data to improve efficiency. However, gathering and centralising such data is both logistically challenging and costly. Federated AI overcomes these challenges by enabling distributed data processing. Here’s why this matters:
Data Privacy and Security: By keeping data on-site and only sharing model parameters, Federated AI protects sensitive building data, addressing privacy concerns while allowing data-informed decision-making. This is crucial in sectors like healthcare, finance, and now, energy management.
Cost-Effectiveness: Centralised data systems can be expensive to maintain, particularly when dealing with multiple building sites. Federated AI reduces data storage needs by distributing the data workload across many sources, allowing efficient and low-cost operations.
Scalability and Adaptability: Each building has unique patterns, architecture, and energy needs. Federated AI allows for personalised learning at each site, capturing these differences while contributing to a general model. This adaptability is especially valuable in non-domestic buildings, which have diverse structures and requirements.
Faster Model Training and Real-Time Updates: Federated AI accelerates model training because insights are generated and shared in real-time. As each building learns and adapts, the main model also improves, leading to faster energy optimisations across the entire building network.
How Optimise-AI Leverages Federated AI
At Optimise-AI, Federated AI is the backbone of our Predict and Optimise solutions, both of which aim to make real-time adjustments to energy usage without compromising data security. Here’s how we do it:
Co-Learning Across Buildings: Each building within an estate runs its own local model, learning from real-time energy patterns and usage behaviors. Through Federated AI, these local models send anonymised updates to a central model, which aggregates the information without needing access to the actual data. This way, the model becomes more robust with each learning cycle, benefiting from co-learning across multiple buildings.
Adapting to Diverse Needs: Because Federated AI allows each building to learn individually, it’s possible to account for distinct features like building materials, weather patterns, and occupancy rates. These insights are then shared back to the broader model, enhancing the Predict tool’s accuracy across diverse environments.
Real-Time Interventions: By leveraging Federated AI, Optimise-AI's digital twin technology, which includes 'Predict’ and ‘Optimise’, can make timely adjustments for each site, minimising energy waste and increasing savings without delays.
Future Prospects: Federated AI and the Decentralised Energy Grid
Federated AI has the potential to play a critical role in the decentralised energy grid of the future, where energy production and consumption are more localised and dynamically managed. In such a grid, Federated AI could be used to aggregate data from local renewable sources, such as solar panels or wind turbines, helping balance supply and demand in real-time.
In summary, Federated AI provides a framework that brings scalability, security, and adaptability to the building energy management sector. Optimise-AI leverages this technology to address the unique energy needs of each building while enhancing our understanding of energy usage patterns across entire estates. As we continue on the path toward decarbonisation, Federated AI will likely become indispensable, allowing us to use energy more efficiently, save costs, and contribute to a more sustainable future.
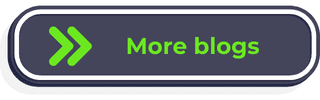
Copyright ©
2025
optimise-ai.com






Back to Blog
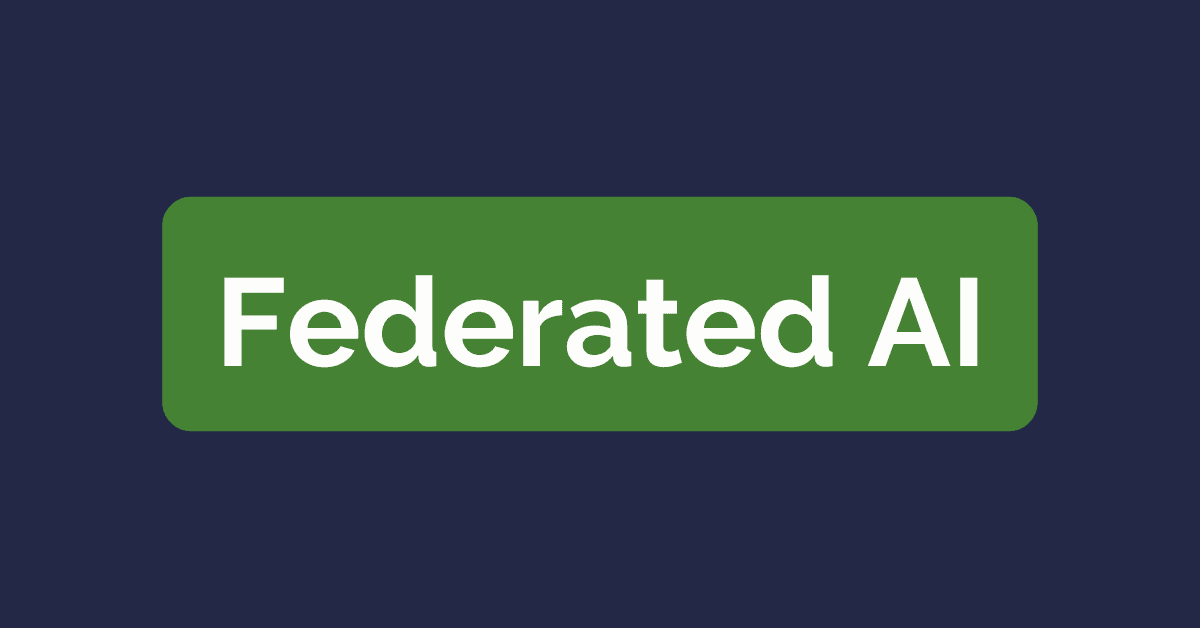
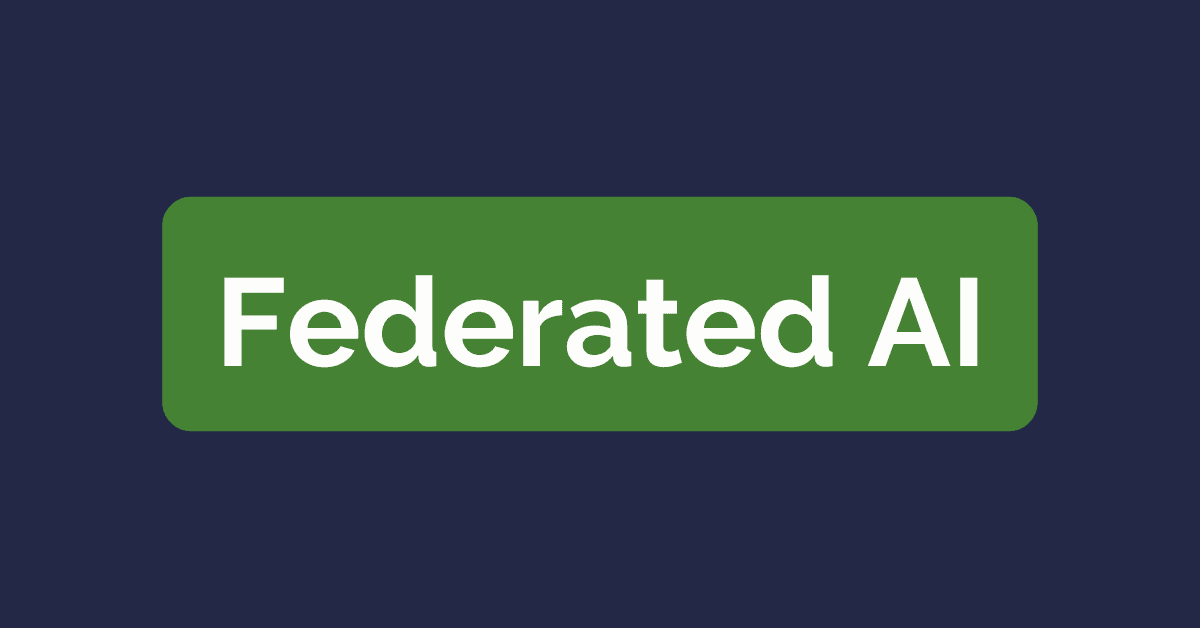
What is Federated AI, and How Is It Shaping the Future of Building Energy Management?
Federated AI is a transformative approach to machine learning that’s gaining traction across industries, especially in fields that require secure, scalable data analysis. Within building energy management, Federated AI is a game changer, allowing systems like Optimise-AI’s Predict and Optimise tools to learn from multiple buildings’ energy data without needing centralised storage. This decentralisation opens new possibilities for energy management, all while preserving data privacy and enhancing adaptability. But what exactly is Federated AI, and why is it so valuable for creating energy-efficient, sustainable buildings?
What Is Federated AI?
Federated AI, also known as Federated Learning (FL), is a machine learning framework where multiple devices or data sources collaborate to train an AI model, all while keeping data localised. Instead of gathering all data on a central server, Federated AI enables individual devices or nodes to learn independently and share only their learned insights (model parameters) with a central model.
For example, imagine multiple buildings, each equipped with an HVAC system, contributing anonymised insights about energy consumption patterns without transferring actual data. Each building "learns" on its own, and only the model updates are shared to improve overall system accuracy. This approach allows learning from diverse data sources while protecting each source’s privacy and confidentiality.
Why Is Federated AI Valuable for Energy Management?
Building energy optimisation often requires vast amounts of data to improve efficiency. However, gathering and centralising such data is both logistically challenging and costly. Federated AI overcomes these challenges by enabling distributed data processing. Here’s why this matters:
Data Privacy and Security: By keeping data on-site and only sharing model parameters, Federated AI protects sensitive building data, addressing privacy concerns while allowing data-informed decision-making. This is crucial in sectors like healthcare, finance, and now, energy management.
Cost-Effectiveness: Centralised data systems can be expensive to maintain, particularly when dealing with multiple building sites. Federated AI reduces data storage needs by distributing the data workload across many sources, allowing efficient and low-cost operations.
Scalability and Adaptability: Each building has unique patterns, architecture, and energy needs. Federated AI allows for personalised learning at each site, capturing these differences while contributing to a general model. This adaptability is especially valuable in non-domestic buildings, which have diverse structures and requirements.
Faster Model Training and Real-Time Updates: Federated AI accelerates model training because insights are generated and shared in real-time. As each building learns and adapts, the main model also improves, leading to faster energy optimisations across the entire building network.
How Optimise-AI Leverages Federated AI
At Optimise-AI, Federated AI is the backbone of our Predict and Optimise solutions, both of which aim to make real-time adjustments to energy usage without compromising data security. Here’s how we do it:
Co-Learning Across Buildings: Each building within an estate runs its own local model, learning from real-time energy patterns and usage behaviors. Through Federated AI, these local models send anonymised updates to a central model, which aggregates the information without needing access to the actual data. This way, the model becomes more robust with each learning cycle, benefiting from co-learning across multiple buildings.
Adapting to Diverse Needs: Because Federated AI allows each building to learn individually, it’s possible to account for distinct features like building materials, weather patterns, and occupancy rates. These insights are then shared back to the broader model, enhancing the Predict tool’s accuracy across diverse environments.
Real-Time Interventions: By leveraging Federated AI, Optimise-AI's digital twin technology, which includes 'Predict’ and ‘Optimise’, can make timely adjustments for each site, minimising energy waste and increasing savings without delays.
Future Prospects: Federated AI and the Decentralised Energy Grid
Federated AI has the potential to play a critical role in the decentralised energy grid of the future, where energy production and consumption are more localised and dynamically managed. In such a grid, Federated AI could be used to aggregate data from local renewable sources, such as solar panels or wind turbines, helping balance supply and demand in real-time.
In summary, Federated AI provides a framework that brings scalability, security, and adaptability to the building energy management sector. Optimise-AI leverages this technology to address the unique energy needs of each building while enhancing our understanding of energy usage patterns across entire estates. As we continue on the path toward decarbonisation, Federated AI will likely become indispensable, allowing us to use energy more efficiently, save costs, and contribute to a more sustainable future.
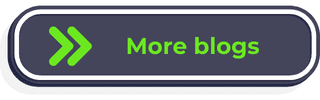
Copyright ©
2025
optimise-ai.com
Copyright ©
2025
optimise-ai.com






Back to Blog
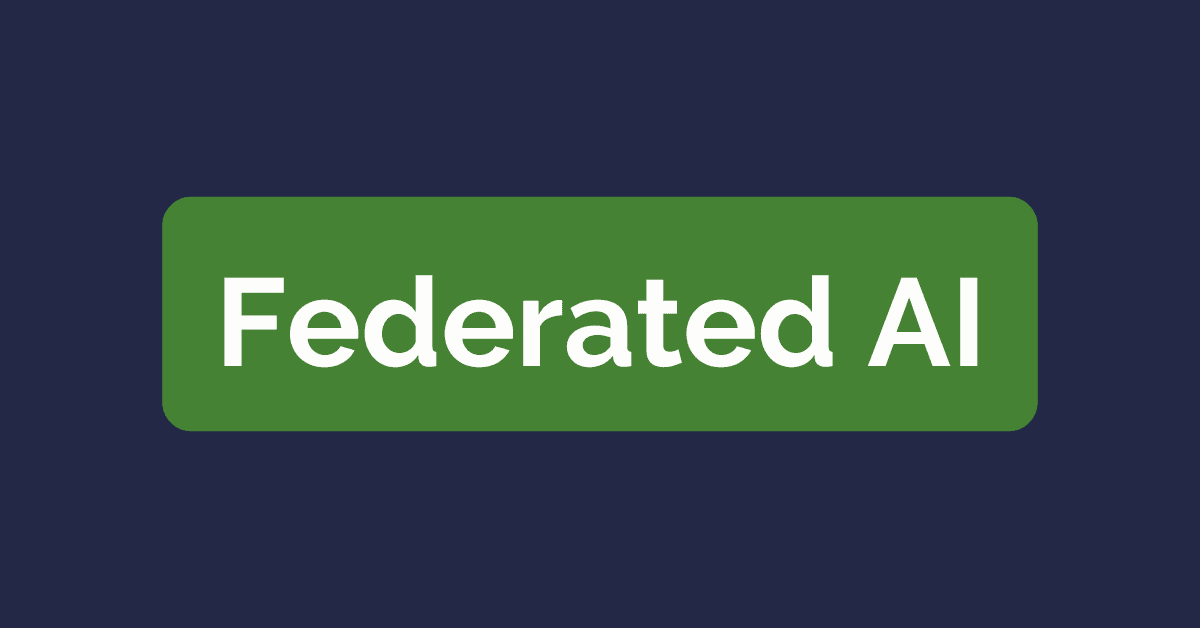
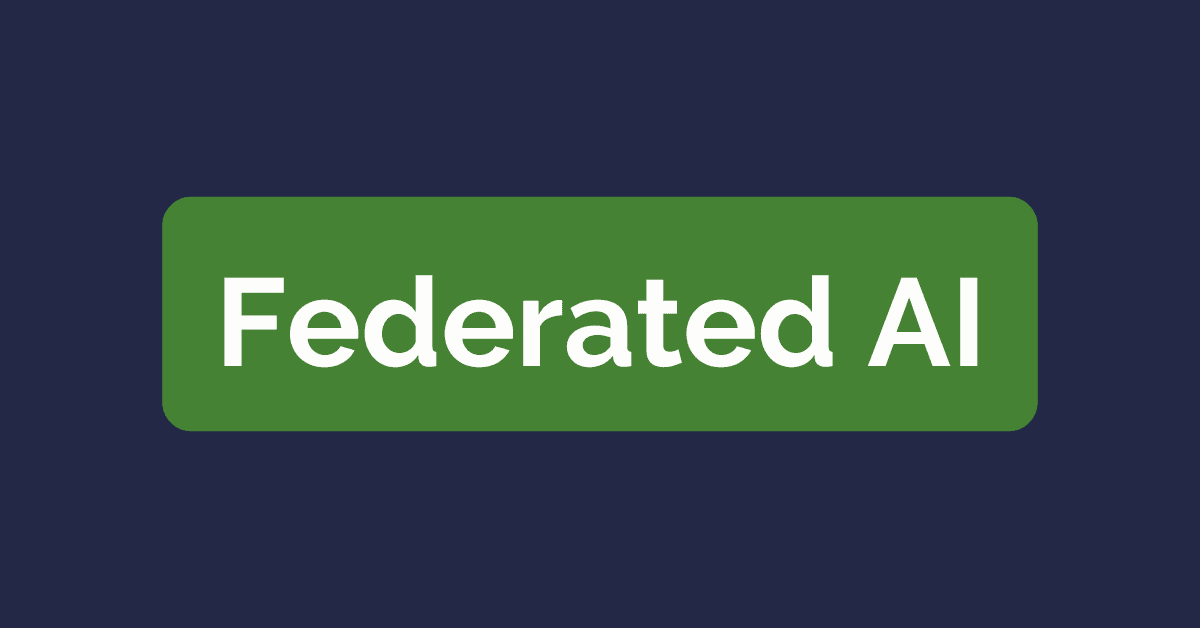
What is Federated AI, and How Is It Shaping the Future of Building Energy Management?
Federated AI is a transformative approach to machine learning that’s gaining traction across industries, especially in fields that require secure, scalable data analysis. Within building energy management, Federated AI is a game changer, allowing systems like Optimise-AI’s Predict and Optimise tools to learn from multiple buildings’ energy data without needing centralised storage. This decentralisation opens new possibilities for energy management, all while preserving data privacy and enhancing adaptability. But what exactly is Federated AI, and why is it so valuable for creating energy-efficient, sustainable buildings?
What Is Federated AI?
Federated AI, also known as Federated Learning (FL), is a machine learning framework where multiple devices or data sources collaborate to train an AI model, all while keeping data localised. Instead of gathering all data on a central server, Federated AI enables individual devices or nodes to learn independently and share only their learned insights (model parameters) with a central model.
For example, imagine multiple buildings, each equipped with an HVAC system, contributing anonymised insights about energy consumption patterns without transferring actual data. Each building "learns" on its own, and only the model updates are shared to improve overall system accuracy. This approach allows learning from diverse data sources while protecting each source’s privacy and confidentiality.
Why Is Federated AI Valuable for Energy Management?
Building energy optimisation often requires vast amounts of data to improve efficiency. However, gathering and centralising such data is both logistically challenging and costly. Federated AI overcomes these challenges by enabling distributed data processing. Here’s why this matters:
Data Privacy and Security: By keeping data on-site and only sharing model parameters, Federated AI protects sensitive building data, addressing privacy concerns while allowing data-informed decision-making. This is crucial in sectors like healthcare, finance, and now, energy management.
Cost-Effectiveness: Centralised data systems can be expensive to maintain, particularly when dealing with multiple building sites. Federated AI reduces data storage needs by distributing the data workload across many sources, allowing efficient and low-cost operations.
Scalability and Adaptability: Each building has unique patterns, architecture, and energy needs. Federated AI allows for personalised learning at each site, capturing these differences while contributing to a general model. This adaptability is especially valuable in non-domestic buildings, which have diverse structures and requirements.
Faster Model Training and Real-Time Updates: Federated AI accelerates model training because insights are generated and shared in real-time. As each building learns and adapts, the main model also improves, leading to faster energy optimisations across the entire building network.
How Optimise-AI Leverages Federated AI
At Optimise-AI, Federated AI is the backbone of our Predict and Optimise solutions, both of which aim to make real-time adjustments to energy usage without compromising data security. Here’s how we do it:
Co-Learning Across Buildings: Each building within an estate runs its own local model, learning from real-time energy patterns and usage behaviors. Through Federated AI, these local models send anonymised updates to a central model, which aggregates the information without needing access to the actual data. This way, the model becomes more robust with each learning cycle, benefiting from co-learning across multiple buildings.
Adapting to Diverse Needs: Because Federated AI allows each building to learn individually, it’s possible to account for distinct features like building materials, weather patterns, and occupancy rates. These insights are then shared back to the broader model, enhancing the Predict tool’s accuracy across diverse environments.
Real-Time Interventions: By leveraging Federated AI, Optimise-AI's digital twin technology, which includes 'Predict’ and ‘Optimise’, can make timely adjustments for each site, minimising energy waste and increasing savings without delays.
Future Prospects: Federated AI and the Decentralised Energy Grid
Federated AI has the potential to play a critical role in the decentralised energy grid of the future, where energy production and consumption are more localised and dynamically managed. In such a grid, Federated AI could be used to aggregate data from local renewable sources, such as solar panels or wind turbines, helping balance supply and demand in real-time.
In summary, Federated AI provides a framework that brings scalability, security, and adaptability to the building energy management sector. Optimise-AI leverages this technology to address the unique energy needs of each building while enhancing our understanding of energy usage patterns across entire estates. As we continue on the path toward decarbonisation, Federated AI will likely become indispensable, allowing us to use energy more efficiently, save costs, and contribute to a more sustainable future.
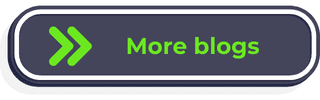
Copyright ©
2025
optimise-ai.com
Copyright ©
2025
optimise-ai.com